Introduction to Artificial intelligence
Table of Contents
History of Artificial intelligence
Artificial Intelligence is not a new word and not a new technology for researchers. This technology is much older than you would imagine. Even there are the myths of Mechanical men in Ancient Greek and Egyptian Myths. Following are some milestones in the history of AI which defines the journey from the AI generation to till date development.
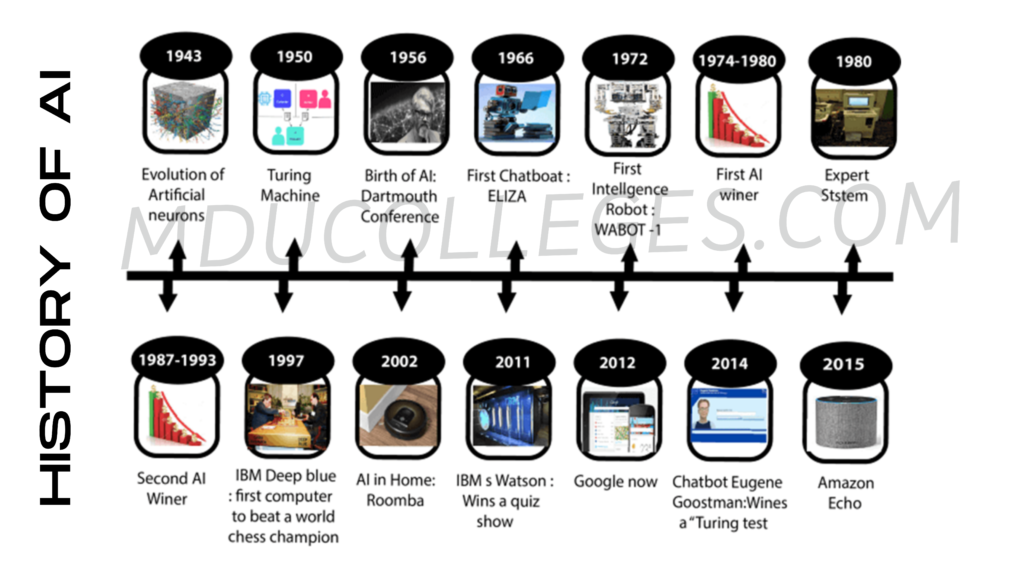
WHAT IS ARTIFICAL INTELLIGENCE?
Artificial Intelligence is composed of two words Artificial and Intelligence, where Artificial defines “man-made,” and intelligence defines “thinking power”, hence AI means “a man-made thinking power. According to the father of Artificial Intelligence, John McCarthy, it is The science and engineering of making intelligent machines, especially intelligent computer programs
“It is a branch of computer science by which we can create intelligent machines which can behave like a human, think like humans, and able to make decisions.”
Artificial Intelligence is a way of making a computer, a computer-controlled robot, or a software think intelligently, in a similar manner the intelligent humans think.
Artificial Intelligence exists when a machine can have human based skills such as learning, reasoning, and solving problems With Artificial Intelligence you do not need to pre program a machine to do some work, despite that you can create a machine with programmed algorithms which can work with own intelligence, and that is the awesomeness of AI. AI is one of the fascinating and universal fields of Computer science which has a great scope in future. AI holds a tendency to cause a machine to work as a human. one of the booming technologies of computer science is Artificial Intelligence which is ready to create a new revolution in the world by making intelligent machines.The Artificial Intelligence is now all around us. It is currently working with a variety of subfields, ranging from general to specific, such as self-driving cars, playing chess, proving theorems, playing music, Painting, etc.
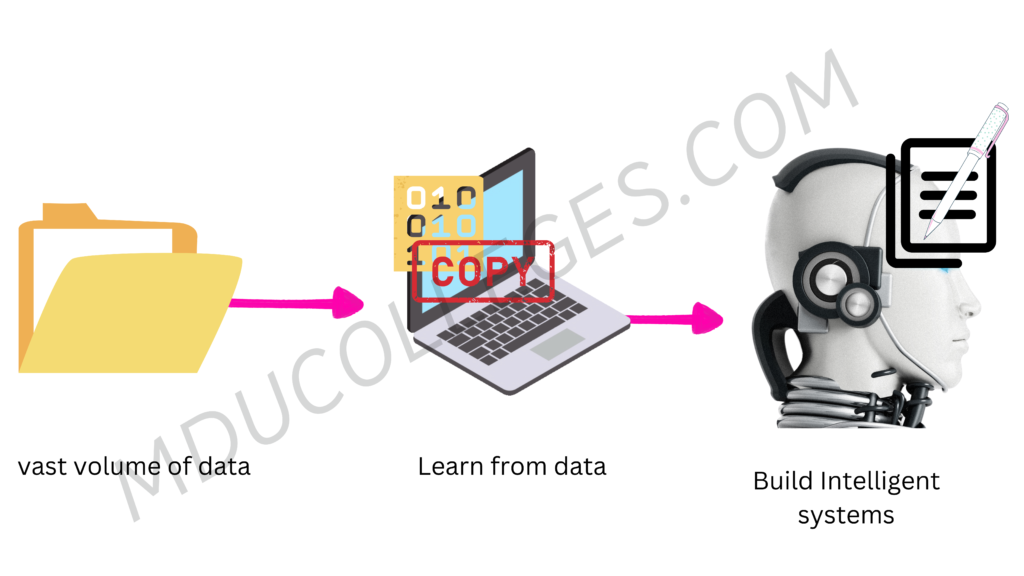
Definition of Intelligence with the scope of AI keeping in mind
1.) Ability to take decisions:
The ability to make complex decisions from a set of factors that make a large number of decision-makers. Making the optimum, accurate and accurate decision is very important. It measures your intelligence in general terms as well as in the context of AI.
2.) Ability to prove results:
Once you make a decision, then the ability to prove that the decision has been chosen, it matters a lot, and in this way this factor also measures the intellect.
3.) Ability to think logically:
There are many things in the world, which can’t be proven by a mathematical formulae or proof, instead you apply your common sense, think logically and come to a conclusion. This ability to think logically and apply common sense also measures intellect.
4.) Ability to learn and improve:
Every time we learn something new, we develop our experiences and these experiences help us in making better decisions and better opportunities in the future.
In the context of AI and generic, it is similar in the case of intelligence.The more you learn from the external environment, you should have the ability to get some new information every time.
Therefore, the factors above mentioned will get your wisdom criteria. Apart from this, research and work is going on in the field of AI, in which they are trying to increase the intelligence of machines and systems which can be better decision, give better reasoning, think more logically and learn from the environment.
AI is now being used in almost every sector of business:
- Transportation
- Healthcare
- Banking
- Retail
- Enterainment
- E-commerce
Comprises to Artificial Intelligence:-
Artificial Intelligence is not just a part of computer science even it’s so vast and requires lots of other factors which can contribute to it. To create the AI first we should know that how intelligence is composed, so the Intelligence is an intangible part of our brain which is a combination of Reasoning, learning, problem-solving perception, language understanding, etc.
To achieve the above factors for a machine or software Artificial Intelligence requires the following discipline:
- Mathematics
- Biology
- Psychology
- Sociology
- Computer Science
- Neurons Study
- Statistics
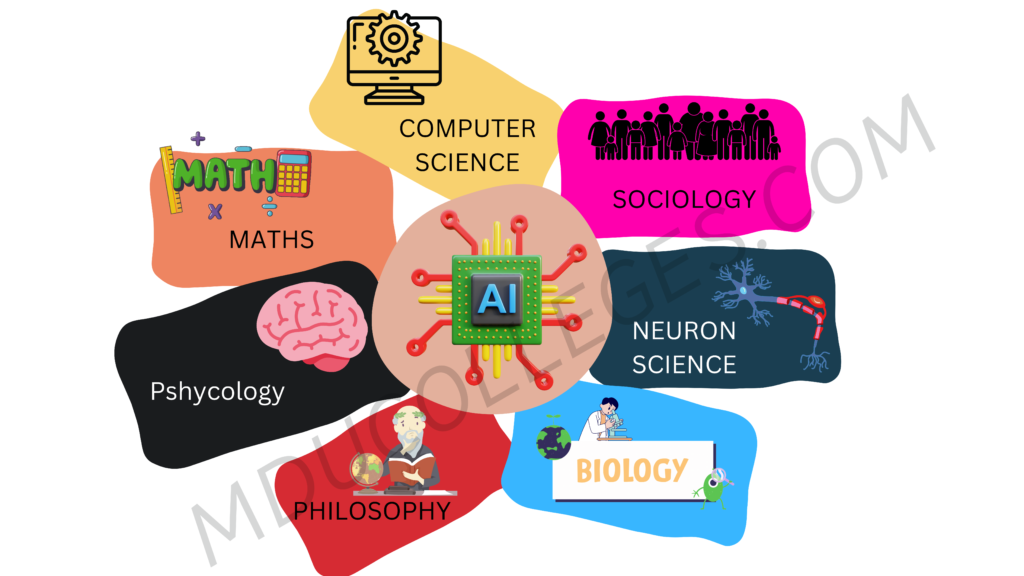
Advantages of Artificial Intelligence
Following are some main advantages of Artificial Intelligence:
- High Accuracy with less errors: AI machines or systems are prone to less errors and high accuracy as it takes decisions as per pre-experience or information.
- High-Speed: AI systems can be of very high-speed and fast-decision making, because of that AI systems can beat a chess champion in the Chess game.
- High reliability:AI machines are highly reliable and can perform the same action multiple times with high accuracy.
- Useful for risky areas: AI machines can be helpful in situations such as defusing a bomb, exploring the ocean floor, where to employ a human can be risky.
- Digital Assistant:AI can be very useful to provide digital assistant to the users such as AI technology is currently used by various E-commerce websites to show the products as per customer requirement.
- Useful as a public utility: AI can be very useful for public utilities such as a self-driving car which can make our journey safer and hassle-free, facial recognition for security purpose, Natural language processing to communicate with the human in human-language, etc.
Disadvantages of Artificial Intelligence
Every technology has some disadvantages, and thesame goes for Artificial intelligence. Being so advantageous technology still, it has some disadvantages which we need to keep in our mind while creating an AI system. Following are the disadvantages of AI:
- High Cost: The hardware and software requirement of AI is very costly as it requires lots of maintenance to meet current world requirements.
- Can’t think out of the box: Even we are making smarter machines with AI, but still they cannot work out of the box, as the robot will only do that work for which they are trained, or programmed.
- No feelings and emotions: AI machines can be an outstanding performer, but still it does not have the feeling so it cannot make any kind of emotional attachment with human, and may sometime be harmful for users if the proper care is not taken.
- Increase dependency on machines: With the increment of technology, people are getting more dependent on devices and hence they are losing their mental capabilities.
- No Original Creativity: As humans are so creative and can imagine some new ideas but still AI machines cannot beat this power of human intelligence and cannot be creative and imaginative.
Application of Artificial Intelligence
Artificial Intelligence has various applications in today’s society. It is becoming essential for today’s time because it can solve complex problems with an efficient way in multiple industries, such as Healthcare, entertainment, finance, education, etc. AI is making our daily life more comfortable and fast.
Following are some sectors which have the application of Artificial Intelligence:
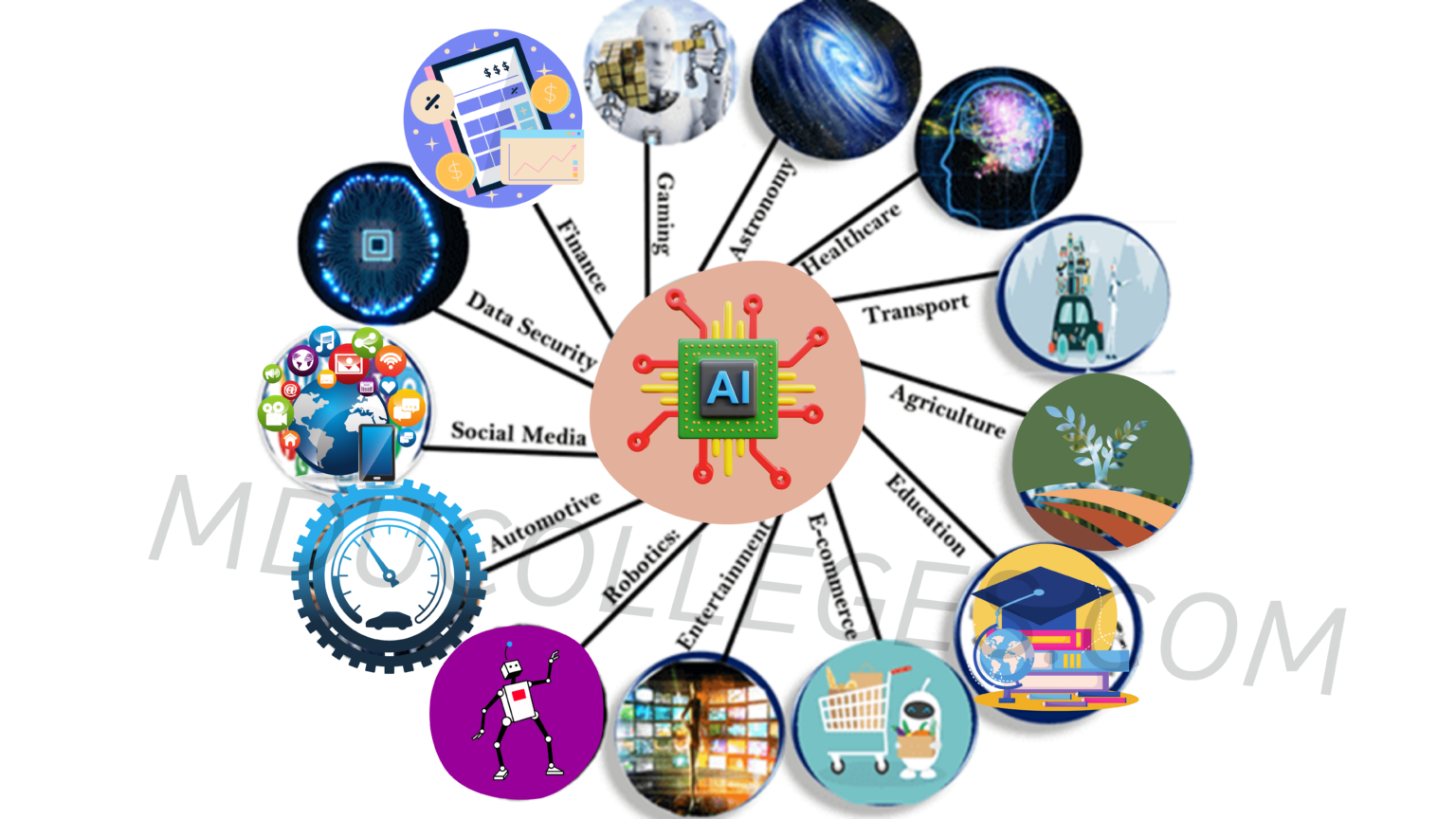
- AI in Astronomy
- Artificial Intelligence can be very useful to solve complex universe problems. AI technology can be helpful for understanding the universe such as how it works, origin, etc.
- AI in Healthcare
- In the last, five to ten years, AI becoming more advantageous for the healthcare industry and going to have a significant impact on this industry.
- Healthcare Industries are applying AI to make a better and faster diagnosis than humans. AI can help doctors with diagnoses and can inform when patients are worsening so that medical help can reach to the patient before hospitalization.
- AI in Gaming
- AI can be used for gaming purpose. The AI machines can play strategic games like chess, where the machine needs to think of a large number of possible places.
- AI in Finance
- AI and finance industries are the best matches for each other. The finance industry is implementing automation, chatbot, adaptive intelligence, algorithm trading, and machine learning into financial processes.
- AI in Data Security
- The security of data is crucial for every company and cyber-attacks are growing very rapidly in the digital world. AI can be used to make your data more safe and secure. Some examples such as AEG bot, AI2 Platform,are used to determine software bug and cyber-attacks in a better way.
- AI in Social Media
- Social Media sites such as Facebook, Twitter, and Snapchat contain billions of user profiles, which need to be stored and managed in a very efficient way. AI can organize and manage massive amounts of data. AI can analyze lots of data to identify the latest trends, hashtag, and requirement of different users.
- AI in Travel & Transport
- AI is becoming highly demanding for travel industries. AI is capable of doing various travel related works such as from making travel arrangement to suggesting the hotels, flights, and best routes to the customers. Travel industries are using AI-powered chatbots which can make human-like interaction with customers for better and fast response.
- AI in Automotive Industry
- Some Automotive industries are using AI to provide virtual assistant to their user for better performance. Such as Tesla has introduced TeslaBot, an intelligent virtual assistant.
- Various Industries are currently working for developing self-driven cars which can make your journey more safe and secure.
- AI in Robotics:
- Artificial Intelligence has a remarkable role in Robotics. Usually, general robots are programmed such that they can perform some repetitive task, but with the help of AI, we can create intelligent robots which can perform tasks with their own experiences without pre-programmed.
- Humanoid Robots are best examples for AI in robotics, recently the intelligent Humanoid robot named as Erica and Sophia has been developed which can talk and behave like humans.
- AI in Entertainment
- We are currently using some AI based applications in our daily life with some entertainment services such as Netflix or Amazon. With the help of ML/AI algorithms, these services show the recommendations for programs or shows.
- AI in Agriculture
- Agriculture is an area which requires various resources, labor, money, and time for best result. Now a day’s agriculture is becoming digital, and AI is emerging in this field. Agriculture is applying AI as agriculture robotics, solid and crop monitoring, predictive analysis. AI in agriculture can be very helpful for farmers.
- AI in E-commerce
- AI is providing a competitive edge to the e-commerce industry, and it is becoming more demanding in the e-commerce business. AI is helping shoppers to discover associated products with recommended size, color, or even brand.
- AI in education:
- AI can automate grading so that the tutor can have more time to teach. AI chatbot can communicate with students as a teaching assistant.
Types of Artificial Intelligence
Artificial Intelligence can be divided in various types, there are mainly two types of main categorization which are based on capabilities and based on functionally of AI. Following is flow diagram which explain the types of AI.
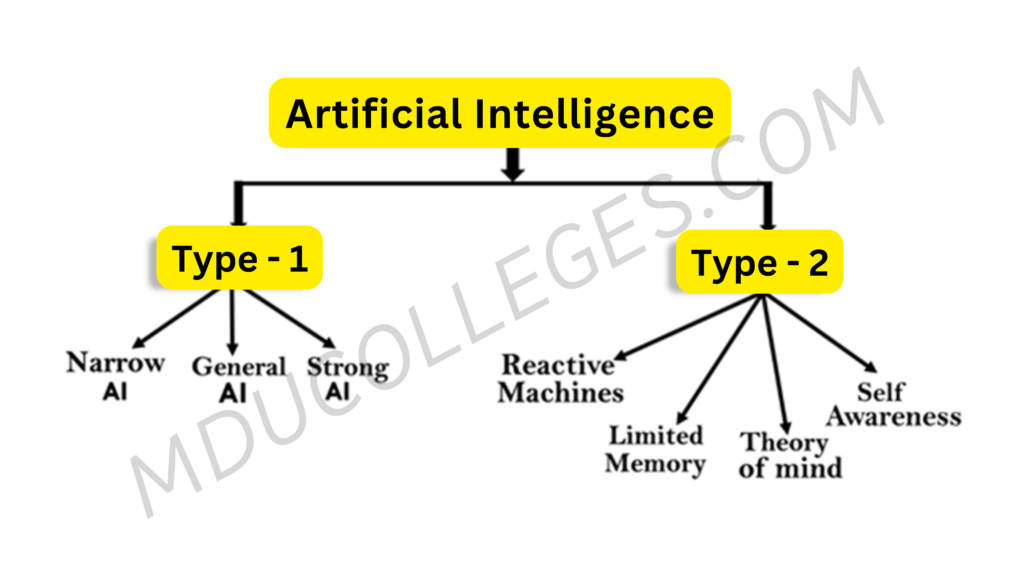
AI type-1: Based on Capabilities
- Weak AI or Narrow AI:
- Narrow AI is a type of AI which is able to perform a dedicated task with intelligence.The most common and currently available AI is Narrow AI in the world of Artificial Intelligence.
- Narrow AI cannot perform beyond its field or limitations, as it is only trained for one specific task. Hence it is also termed as weak AI. Narrow AI can fail in unpredictable ways if it goes beyond its limits.
- Apple Siriis a good example of Narrow AI, but it operates with a limited pre-defined range of functions.
- IBM’s Watson supercomputer also comes under Narrow AI, as it uses an Expert system approach combined with Machine learning and natural language processing.
- Some Examples of Narrow AI are playing chess, purchasing suggestions on e-commerce site, self-driving cars, speech recognition, and image recognition.
- General AI:
- General AI is a type of intelligence which could perform any intellectual task with efficiency like a human.
- The idea behind the general AI to make such a system which could be smarter and think like a human by its own.
- Currently, there is no such system exist which could come under general AI and can perform any task as perfect as a human.
- The worldwide researchers are now focused on developing machines with General AI.
- As systems with general AI are still under research, and it will take lots of efforts and time to develop such systems.
- Super AI:
- Super AI is a level of Intelligence of Systems at which machines could surpass human intelligence, and can perform any task better than human with cognitive properties. It is an outcome of general AI.
- Some key characteristics of strong AI include capability include the ability to think, to reason , solve the puzzle, make judgments, plan, learn, and communicate by its own.
- Super AI is still a hypothetical concept of Artificial Intelligence. Development of such systems in real is still world changing task.
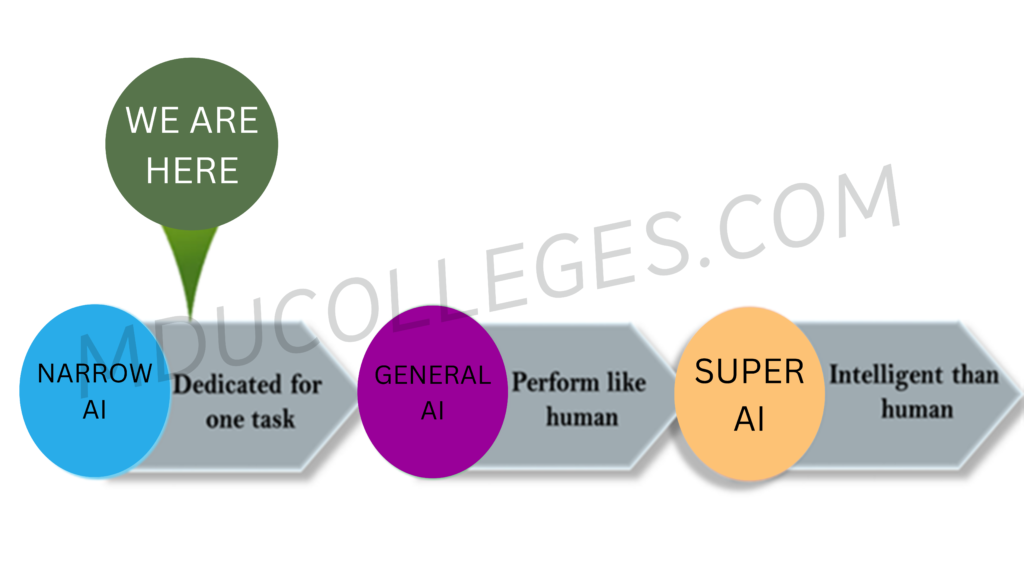
Artificial Intelligence type-2: Based on functionality
- Reactive Machines
- Purely reactive machines are the most basic types of Artificial Intelligence.
- Such AI systems do not store memories or past experiences for future actions.
- These machines only focus on current scenarios and react on it as per possible best action.
- IBM’s Deep Blue system is an example of reactive machines.
- Google’s AlphaGo is also an example of reactive machines.
- Limited Memory
- Limited memory machines can store past experiences or some data for a short period of time.
- These machines can use stored data for a limited time period only.
- Self-driving cars are one of the best examples of Limited Memory systems. These cars can store recent speed of nearby cars, the distance of other cars, speed limit, and other information to navigate the road.
- Theory of Mind
- Theory of Mind AI should understand the human emotions, people, beliefs, and be able to interact socially like humans.
- This type of AI machines are still not developed, but researchers are making lots of efforts and improvement for developing such AI machines.
- Self-Awareness
- Self-awareness AI is the future of Artificial Intelligence. These machines will be super intelligent, and will have their own consciousness, sentiments, and self-awareness.
- These machines will be smarter than human mind.
Self-Awareness AI does not exist in reality still and it is a hypothetical concept.
Search algorithms of Artificial Intelligence
In Artificial Intelligence, Search techniques are universal problem-solving methods. Rational agents or Problem-solving agents in AI mostly used these search strategies or algorithms to solve a specific problem and provide the best result. Problem-solving agents are the goal-based agents and use atomic representation. In this topic, we will learn various problem-solving search algorithms.
Types of Search alogirthms of AI:-
- Uninformed Search
- Informed Search
- Population Based Search
Uninformed Search:-
Uninformed search is a class of general-purpose search algorithms which operates in brute force-way. Uninformed search algorithms do not have additional information about state or search space other than how to traverse the tree, so it is also called blind search.
Following are the various types of uninformed search algorithms:
- Breadth-first Search
- Depth-first Search
- Depth-limited Search
- Iterative deepening depth-first search
- Uniform cost search
- Bidirectional Search
- Breadth-first Search:
Breadth-first search is the most common search strategy for traversing a tree or graph. This algorithm searches breadthwise in a tree or graph, so it is called breadth-first search.
BFS algorithm starts searching from the root node of the tree and expands all successor node at the current level before moving to nodes of next level.
The breadth-first search algorithm is an example of a general-graph search algorithm.
Breadth-first search implemented using FIFO queue data structure.
Advantages:
BFS will provide a solution if any solution exists.
If there are more than one solutions for a given problem, then BFS will provide the minimal solution which requires the least number of steps.
Disadvantages:
It requires lots of memory since each level of the tree must be saved into memory to expand the next level.
BFS needs lots of time if the solution is far away from the root node.
Example:
In the below tree structure, we have shown the traversing of the tree using BFS algorithm from the root node S to goal node K. BFS search algorithm traverse in layers, so it will follow the path which is shown by the dotted arrow, and the traversed path will be:
S—> A—>B—->C—>D—->G—>H—>E—->F—->I—->K
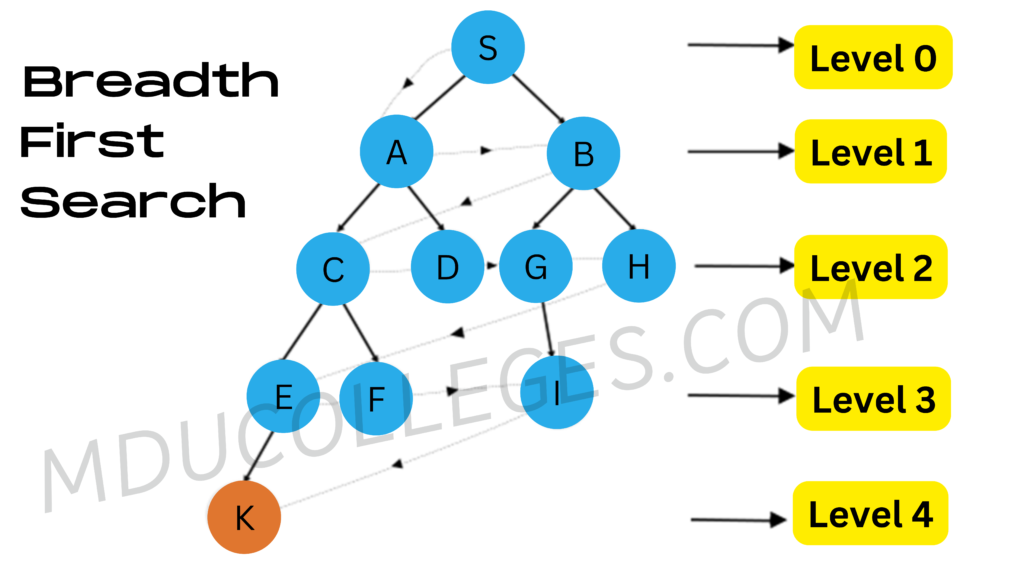
Time Complexity: Time Complexity of BFS algorithm can be obtained by the number of nodes traversed in BFS until the shallowest Node. Where the d= depth of shallowest solution and b is a node at every state.
T (b) = 1+b2+b3+…….+ bd= O (bd)
Space Complexity: Space complexity of BFS algorithm is given by the Memory size of frontier which is O(bd).
Completeness: BFS is complete, which means if the shallowest goal node is at some finite depth, then BFS will find a solution.
Optimality: BFS is optimal if path cost is a non-decreasing function of the depth of the node.
- Depth-first Search
Depth-first search isa recursive algorithm for traversing a tree or graph data structure.
It is called the depth-first search because it starts from the root node and follows each path to its greatest depth node before moving to the next path.
DFS uses a stack data structure for its implementation.
The process of the DFS algorithm is similar to the BFS algorithm.
Note: Backtracking is an algorithm technique for finding all possible solutions using recursion.
Advantage:
DFS requires very less memory as it only needs to store a stack of the nodes on the path from root node to the current node.
It takes less time to reach to the goal node than BFS algorithm (if it traverses in the right path).
Disadvantage:
There is the possibility that many states keep re-occurring, and there is no guarantee of finding the solution.
DFS algorithm goes for deep down searching and sometime it may go to the infinite loop.
Example:
In the below search tree, we have shown the flow of depth-first search, and it will follow the order as:
Root node—>Left node —-> right node.
It will start searching from root node S, and traverse A, then B, then D and E, after traversing E, it will backtrack the tree as E has no other successor and still goal node is not found. After backtracking it will traverse node C and then G, and here it will terminate as it found goal node.
Completeness: DFS search algorithm is complete within finite state space as it will expand every node within a limited search tree.
Time Complexity: Time complexity of DFS will be equivalent to the node traversed by the algorithm. It is given by:
T(n)= 1+ n2+ n3 +………+ nm=O(nm)
Where, m= maximum depth of any node and this can be much larger than d (Shallowest solution depth)
Space Complexity: DFS algorithm needs to store only single path from the root node, hence space complexity of DFS is equivalent to the size of the fringe set, which is O(bm).
Optimal: DFS search algorithm is non-optimal, as it may generate a large number of steps or high cost to reach to the goal node.
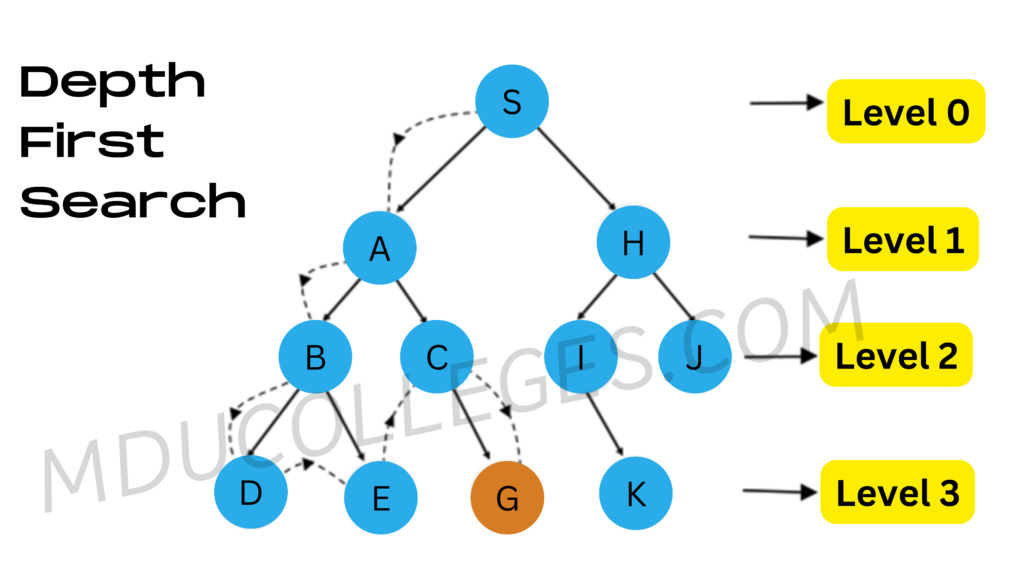
- Depth-Limited Search Algorithm:
A depth-limited search algorithm is similar to depth-first search with a predetermined limit. Depth-limited search can solve the drawback of the infinite path in the Depth-first search. In this algorithm, the node at the depth limit will treat as it has no successor nodes further.
Depth-limited search can be terminated with two Conditions of failure:
Standard failure value: It indicates that problem does not have any solution.
Cutoff failure value: It defines no solution for the problem within a given depth limit.
Advantages:
Depth-limited search is Memory efficient.
Disadvantages:
Depth-limited search also has a disadvantage of incompleteness.
It may not be optimal if the problem has more than one solution.
Example:
Completeness: DLS search algorithm is complete if the solution is above the depth-limit.
Time Complexity: Time complexity of DLS algorithm is O(bℓ).
Space Complexity: Space complexity of DLS algorithm is O(b×ℓ).
Optimal: Depth-limited search can be viewed as a special case of DFS, and it is also not optimal even if ℓ>d.
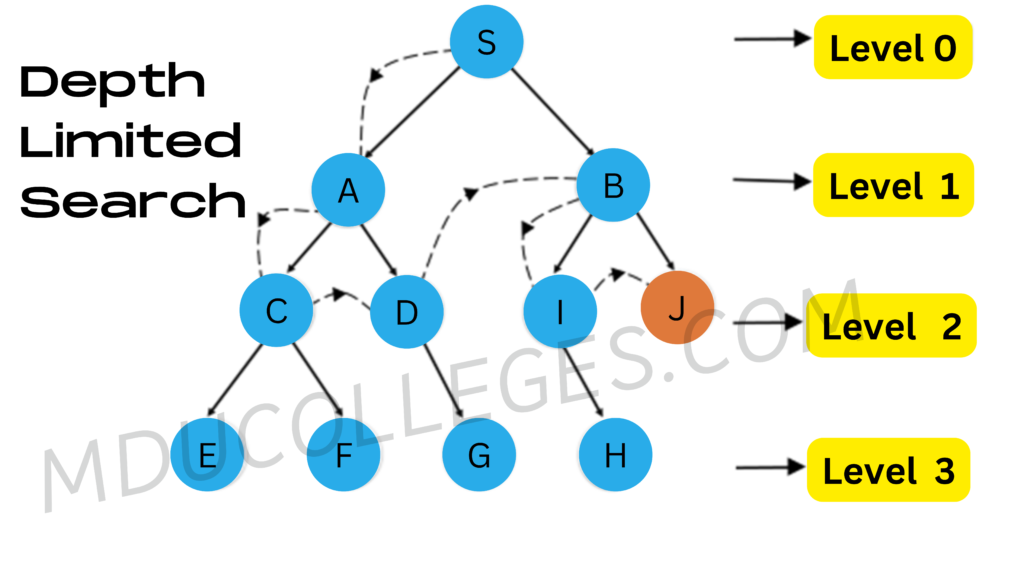
- Uniform-cost Search Algorithm:
Uniform-cost search is a searching algorithm used for traversing a weighted tree or graph. This algorithm comes into play when a different cost is available for each edge. The primary goal of the uniform-cost search is to find a path to the goal node which has the lowest cumulative cost. Uniform-cost search expands nodes according to their path costs form the root node. It can be used to solve any graph/tree where the optimal cost is in demand. A uniform-cost search algorithm is implemented by the priority queue. It gives maximum priority to the lowest cumulative cost. Uniform cost search is equivalent to BFS algorithm if the path cost of all edges is the same.
Advantages:
Uniform cost search is optimal because at every state the path with the least cost is chosen.
Disadvantages:
It does not care about the number of steps involve in searching and only concerned about path cost. Due to which this algorithm may be stuck in an infinite loop.
Example:
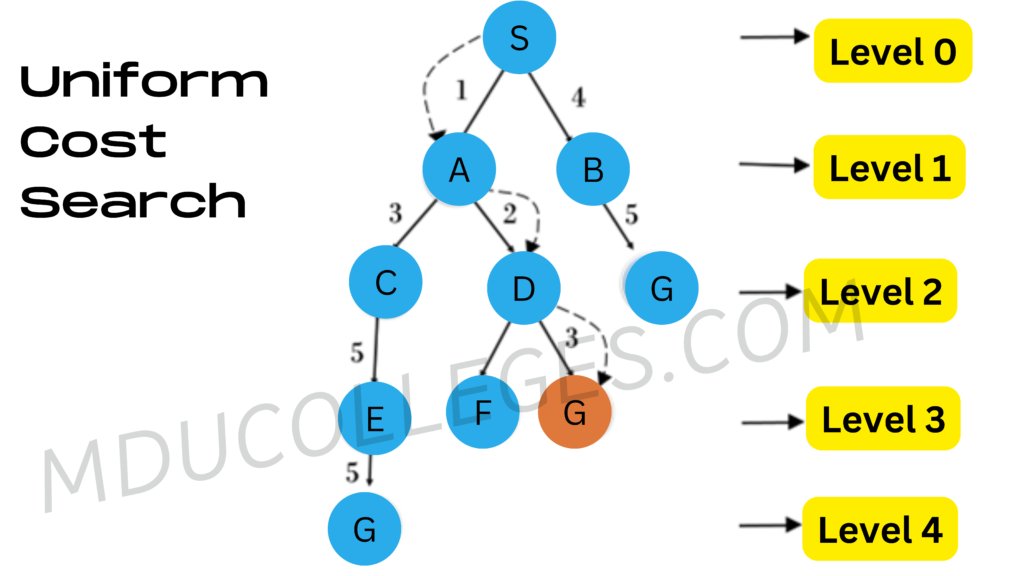
Completeness: Uniform-cost search is complete, such as if there is a solution, UCS will find it.
Time Complexity: Let C* is Cost of the optimal solution, and ε is each step to get closer to the goal node. Then the number of steps is = C*/ε+1. Here we have taken +1, as we start from state 0 and end to C*/ε.
Hence, the worst-case time complexity of Uniform-cost search isO(b1 + [C*/ε])/.
Space Complexity:
The same logic is for space complexity so, the worst-case space complexity of Uniform-cost search is O(b1 + [C*/ε]).
Optimal: Uniform-cost search is always optimal as it only selects a path with the lowest path cost.
- Iterative deepeningdepth-first Search:
The iterative deepening algorithm is a combination of DFS and BFS algorithms. This search algorithm finds out the best depth limit and does it by gradually increasing the limit until a goal is found.
This algorithm performs depth-first search up to a certain “depth limit”, and it keeps increasing the depth limit after each iteration until the goal node is found.
This Search algorithm combines the benefits of Breadth-first search’s fast search and depth-first search’s memory efficiency.
The iterative search algorithm is useful uninformed search when search space is large, and depth of goal node is unknown.
Advantages:
Itcombines the benefits of BFS and DFS search algorithm in terms of fast search and memory efficiency.
Disadvantages:
The main drawback of IDDFS is that it repeats all the work of the previous phase.
Example:
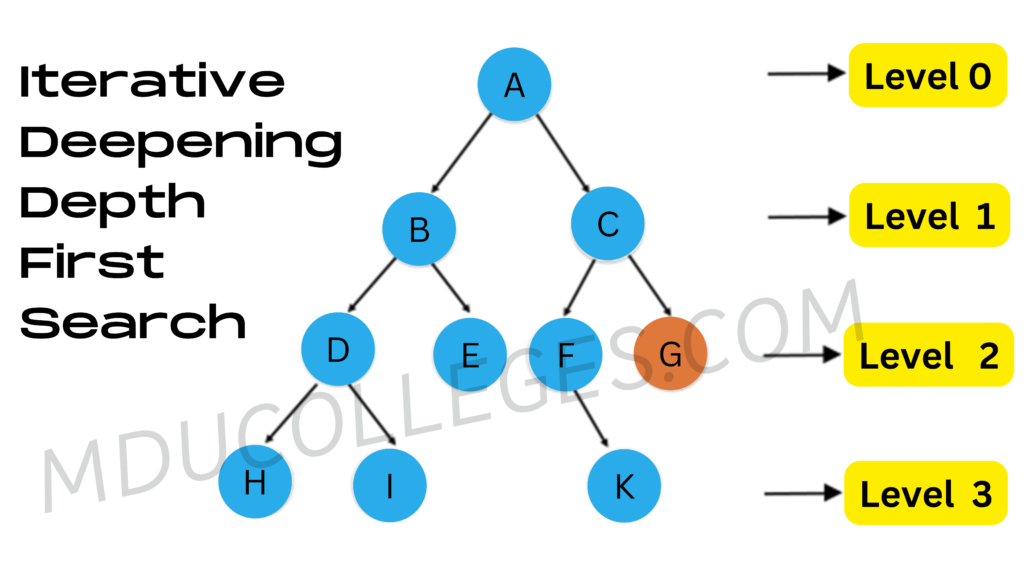
Following tree structure is showing the iterative deepening depth-first search. IDDFS algorithm performs various iterations until it does not find the goal node. The iteration performed by the algorithm is given as:
1’st Iteration—–> A
2’nd Iteration—-> A, B, C
3’rd Iteration——>A, B, D, E, C, F, G
4’th Iteration——>A, B, D, H, I, E, C, F, K, G
In the fourth iteration, the algorithm will find the goal node.
Completeness: This algorithm is complete is ifthe branching factor is finite.
Time Complexity: Let’s suppose b is the branching factor and depth is d then the worst-case time complexity is O(bd).
Space Complexity: The space complexity of IDDFS will be O(bd).
Optimal: IDDFS algorithm is optimal if path cost is a non- decreasing function of the depth of the node.
- Bidirectional Search Algorithm:
Bidirectional search algorithm runs two simultaneous searches, one form initial state called as forward-search and other from goal node called as backward-search, to find the goal node. Bidirectional search replaces one single search graph with two small subgraphs in which one starts the search from an initial vertex and other starts from goal vertex. The search stops when these two graphs intersect each other.
Bidirectional search can use search techniques such as BFS, DFS, DLS, etc.
Advantages:
Bidirectional search is fast.
Bidirectional search requires less memory
Disadvantages:
Implementation of the bidirectional search tree is difficult.
In bidirectional search, one should know the goal state in advance.
Example:
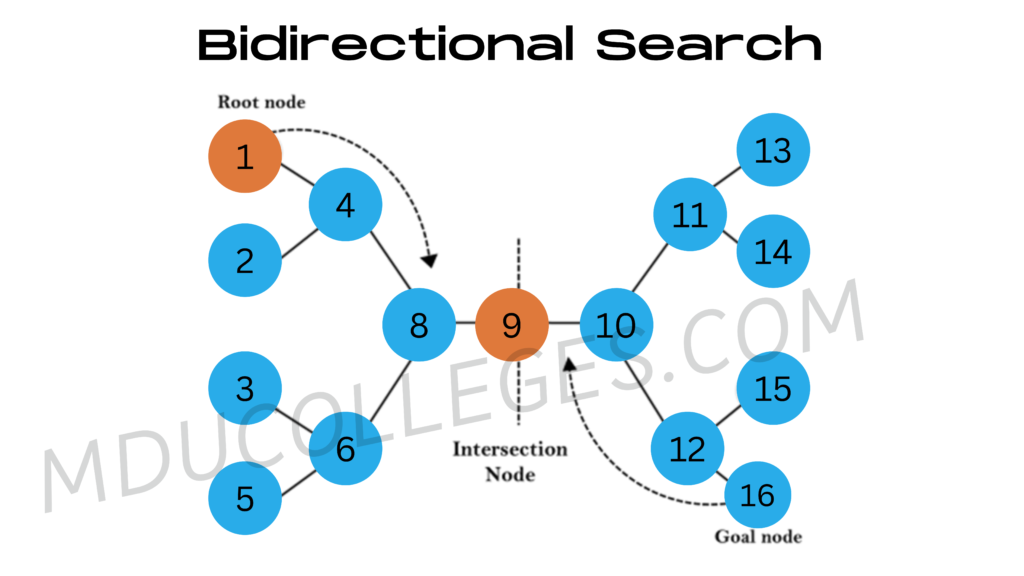
In the below search tree, bidirectional search algorithm is applied. This algorithm divides one graph/tree into two sub-graphs. It starts traversing from node 1 in the forward direction and starts from goal node 16 in the backward direction.
The algorithm terminates at node 9 where two searches meet.
Completeness: Bidirectional Search is complete if we use BFS in both searches.
Time Complexity: Time complexity of bidirectional search using BFS is O(bd).
Space Complexity: Space complexity of bidirectional search is O(bd).
Optimal: Bidirectional search is Optimal.
Informed Search Algorithm (Heuristics Function)
So far we have talked about the uninformed search algorithms which looked through search space for all possible solutions of the problem without having any additional knowledge about search space. But informed search algorithm contains an array of knowledge such as how far we are from the goal, path cost, how to reach to goal node, etc. This knowledge help agents to explore less to the search space and find more efficiently the goal node.The informed search algorithm is more useful for large search space. Informed search algorithm uses the idea of heuristic, so it is also called Heuristic search.
Heuristics function: Heuristic is a function which is used in Informed Search, and it finds the most promising path. It takes the current state of the agent as its input and produces the estimation of how close agent is from the goal. The heuristic method, however, might not always give the best solution, but it guaranteed to find a good solution in reasonable time. Heuristic function estimates how close a state is to the goal. It is represented by h(n), and it calculates the cost of an optimal path between the pair of states. The value of the heuristic function is always positive.
In the informed search we will discuss two main algorithms which are given below:
- Best First Search Algorithm(Greedy search)
- A* Search Algorithm
1.) Best-first Search Algorithm (Greedy Search):
Greedy best-first search algorithm always selects the path which appears best at that moment. It is the combination of depth-first search and breadth-first search algorithms. It uses the heuristic function and search. Best-first search allows us to take the advantages of both algorithms. With the help of best-first search, at each step, we can choose the most promising node. In the best first search algorithm, we expand the node which is closest to the goal node and the closest cost is estimated by heuristic function, i.e.
- f(n)= g(n).
Were, h(n)= estimated cost from node n to the goal.
The greedy best first algorithm is implemented by the priority queue.
Best first search algorithm:
- Step 1: Place the starting node into the OPEN list.
- Step 2: If the OPEN list is empty, Stop and return failure.
- Step 3: Remove the node n, from the OPEN list which has the lowest value of h(n), and places it in the CLOSED list.
- Step 4: Expand the node n, and generate the successors of node n.
- Step 5: Check each successor of node n, and find whether any node is a goal node or not. If any successor node is goal node, then return success and terminate the search, else proceed to Step 6.
- Step 6: For each successor node, algorithm checks for evaluation function f(n), and then check if the node has been in either OPEN or CLOSED list. If the node has not been in both list, then add it to the OPEN list.
- Step 7: Return to Step 2.
Advantages:
- Best first search can switch between BFS and DFS by gaining the advantages of both the algorithms.
- This algorithm is more efficient than BFS and DFS algorithms.
Disadvantages:
- It can behave as an unguided depth-first search in the worst case scenario.
- It can get stuck in a loop as DFS.
- This algorithm is not optimal.
Example:
Consider the below search problem, and we will traverse it using greedy best-first search. At each iteration, each node is expanded using evaluation function f(n)=h(n) , which is given in the below table.
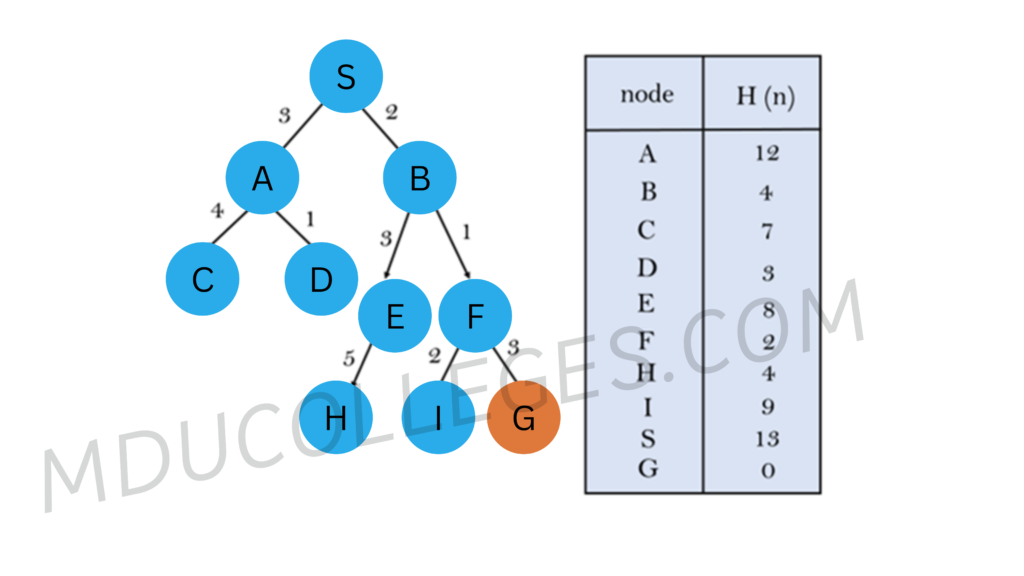
In this search example, we are using two lists which are OPEN and CLOSED Lists. Following are the iteration for traversing the above example.
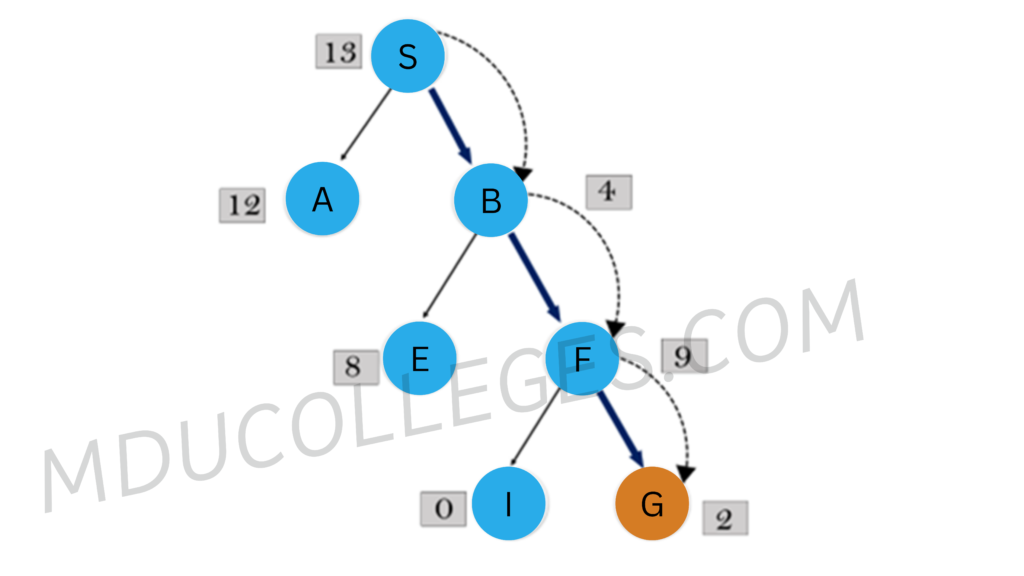
Expand the nodes of S and put in the CLOSED list
Initialization: Open [A, B], Closed [S]
Iteration 1: Open [A], Closed [S, B]
Iteration 2: Open [E, F, A], Closed [S, B]
: Open [E, A], Closed [S, B, F]
Iteration 3: Open [I, G, E, A], Closed [S, B, F]
: Open [I, E, A], Closed [S, B, F, G]
Hence the final solution path will be: S—-> B—–>F—-> G
Time Complexity: The worst case time complexity of Greedy best first search is O(bm).
Space Complexity: The worst case space complexity of Greedy best first search is O(bm). Where, m is the maximum depth of the search space.
Complete: Greedy best-first search is also incomplete, even if the given state space is finite.
Optimal: Greedy best first search algorithm is not optimal.
2.) A* Search Algorithm:
A* search is the most commonly known form of best-first search. It uses heuristic function h(n), and cost to reach the node n from the start state g(n). It has combined features of UCS and greedy best-first search, by which it solve the problem efficiently. A* search algorithm finds the shortest path through the search space using the heuristic function. This search algorithm expands less search tree and provides optimal result faster. A* algorithm is similar to UCS except that it uses g(n)+h(n) instead of g(n).
In A* search algorithm, we use search heuristic as well as the cost to reach the node. Hence we can combine both costs as following, and this sum is called as a fitness number.
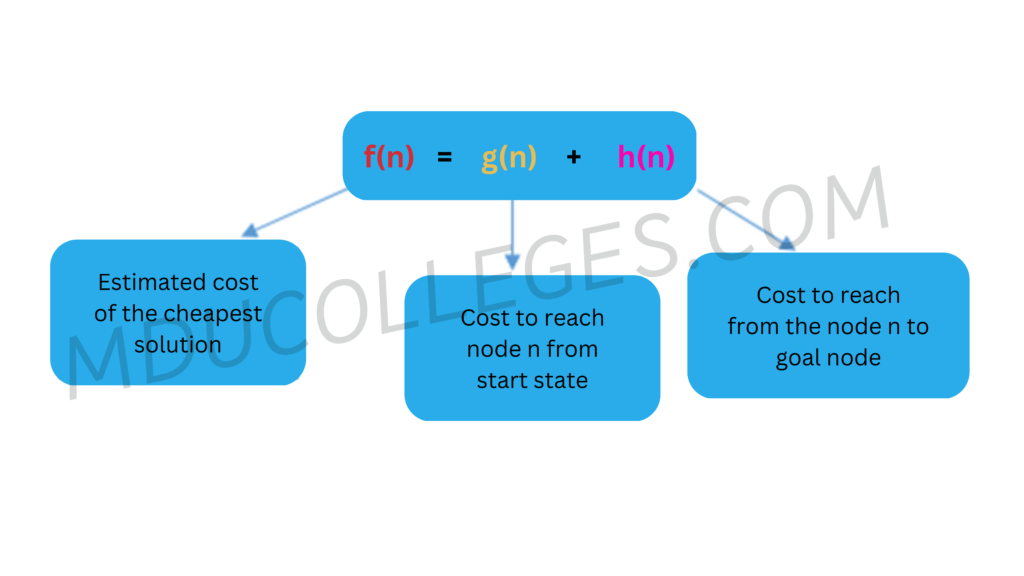
At each point in the search space, only those node is expanded which have the lowest value of f(n), and the algorithm terminates when the goal node is found.
Algorithm of A* search:
Step1: Place the starting node in the OPEN list.
Step 2: Check if the OPEN list is empty or not, if the list is empty then return failure and stops.
Step 3: Select the node from the OPEN list which has the smallest value of evaluation function (g+h), if node n is goal node then return success and stop, otherwise
Step 4: Expand node n and generate all of its successors, and put n into the closed list. For each successor n’, check whether n’ is already in the OPEN or CLOSED list, if not then compute evaluation function for n’ and place into Open list.
Step 5: Else if node n’ is already in OPEN and CLOSED, then it should be attached to the back pointer which reflects the lowest g(n’) value.
Step 6: Return to Step 2.
Advantages:
A* search algorithm is the best algorithm than other search algorithms.
A* search algorithm is optimal and complete.
This algorithm can solve very complex problems.
Disadvantages:
It does not always produce the shortest path as it mostly based on heuristics and approximation.
A* search algorithm has some complexity issues.
The main drawback of A* is memory requirement as it keeps all generated nodes in the memory, so it is not practical for various large-scale problems.
Example:
In this example, we will traverse the given graph using the A* algorithm. The heuristic value of all states is given in the below table so we will calculate the f(n) of each state using the formula f(n)= g(n) + h(n), where g(n) is the cost to reach any node from start state.
Here we will use OPEN and CLOSED list.
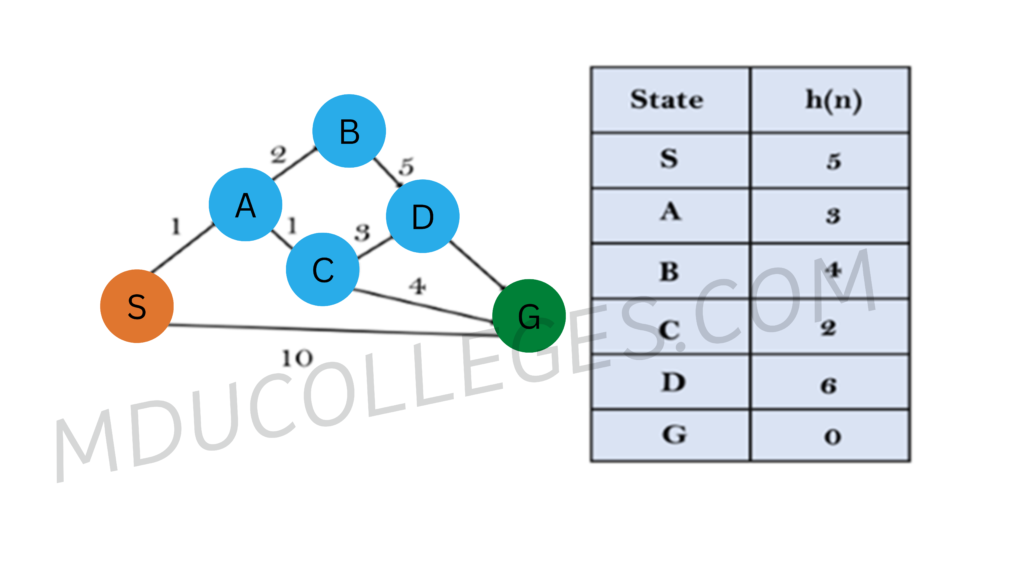
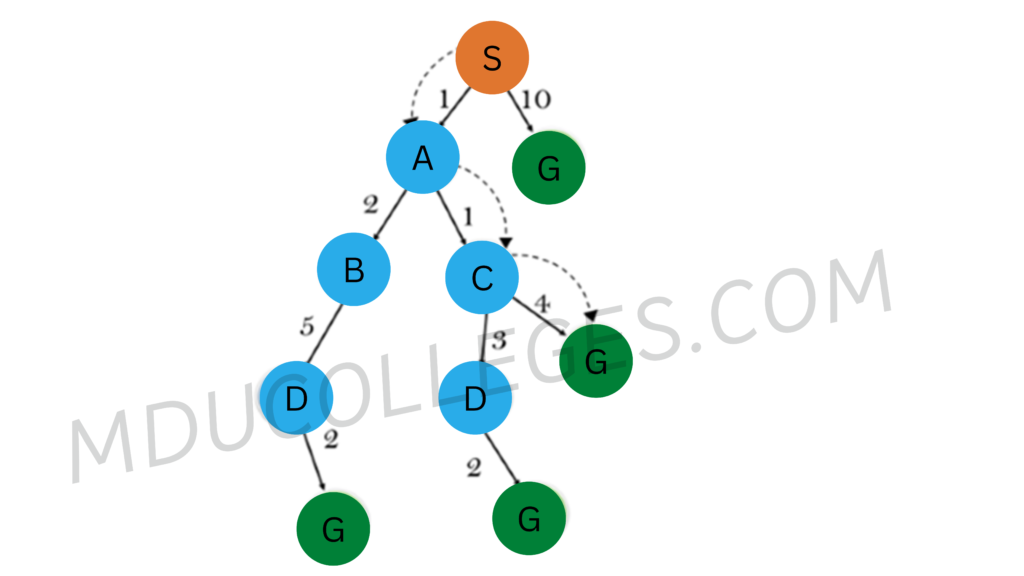
Initialization: {(S, 5)}
Iteration1: {(S–> A, 4), (S–>G, 10)}
Iteration2: {(S–> A–>C, 4), (S–> A–>B, 7), (S–>G, 10)}
Iteration3: {(S–> A–>C—>G, 6), (S–> A–>C—>D, 11), (S–> A–>B, 7), (S–>G, 10)}
Iteration 4 will give the final result, as S—>A—>C—>G it provides the optimal path with cost 6.
Points to remember:
A* algorithm returns the path which occurred first, and it does not search for all remaining paths.
The efficiency of A* algorithm depends on the quality of heuristic.
A* algorithm expands all nodes which satisfy the condition f(n)<=”” li=””>
Complete: A* algorithm is complete as long as:
Branching factor is finite.
Cost at every action is fixed.
Optimal: A* search algorithm is optimal if it follows below two conditions:
Admissible: the first condition requires for optimality is that h(n) should be an admissible heuristic for A* tree search. An admissible heuristic is optimistic in nature.
Consistency: Second required condition is consistency for only A* graph-search.
If the heuristic function is admissible, then A* tree search will always find the least cost path.
Time Complexity: The time complexity of A* search algorithm depends on heuristic function, and the number of nodes expanded is exponential to the depth of solution d. So the time complexity is O(b^d), where b is the branching factor.
Space Complexity: The space complexity of A* search algorithm is O(b^d)
Local Search : Hill Climbing
Hill Climbing is a heuristic search used for mathematical optimization problems in the field of Artificial Intelligence.
Given a large set of inputs and a good heuristic function, it tries to find a sufficiently good solution to the problem. This solution may not be the global optimal maximum. In the above definition, mathematical optimization problems imply that hill-climbing solves the problems where we need to inputs. Example- where we need to minimize the distance traveled by the salesman.
‘Heuristic search’ means that this search algorithm may not find the optimal solution to the problem. However, it will give a good solution in a reasonable time.
Types of Hill Climbing
- Simple Hill climbing:
It examines the neighboring nodes one by one and selects the first neighboring node which optimizes the current cost as the next node.
Algorithm for Simple Hill climbing :
- Evaluate the initial state. If it is a goal state then stop and return success. Otherwise, make the initial state as the current state.
- Loop until the solution state is found or there are no new operators present which can be applied to the current state.
- Select a state that has not been yet applied to the current state and apply it to produce a new state.
- Perform these to evaluate the new state.
- If the current state is a goal state, then stop and return success.
- If it is better than the current state, then make it the current state and proceed further.
- If it is not better than the current state, then continue in the loop until a solution is found.
- Exit from the function.
- Steepest-Ascent Hill climbing:
It first examines all the neighboring nodes and then selects the node closest to the solution state as of the next node.
Algorithm for Steepest Ascent Hill climbing :
- Evaluate the initial state. If it is a goal state then stop and return success. Otherwise, make the initial state as the current state.
- Repeat these steps until a solution is found or the current state does not change
- Select a state that has not been yet applied to the current state.
- Initialize a new ‘best state’ equal to the current state and apply it to produce a new state.
- Perform these to evaluate the new state
- If the current state is a goal state, then stop and return success.
- If it is better than the best state, then make it the best state else continue the loop with another new state.
- Make the best state as the current state and go to Step 2 of the second point.
- Exit from the function.
- Stochastic hill climbing:
It does not examine all the neighboring nodes before deciding which node to select. It just selects a neighboring node at random and decides (based on the amount of improvement in that neighbor) whether to move to that neighbor or to examine another.
- Evaluate the initial state. If it is a goal state then stop and return success. Otherwise, make the initial state the current state.
- Repeat these steps until a solution is found or the current state does not change.
- Select a state that has not been yet applied to the current state.
- Apply the successor function to the current state and generate all the neighbor states.
- Among the generated neighbor states which are better than the current state choose a state randomly (or based on some probability function).
- If the chosen state is the goal state, then return success, else make it the current state and repeat step 2 of the second point.
- Exit from the function
Features of Hill Climbing
- Variant of generating and test algorithm: It is a variant of generating and testing algorithms. The generate and test algorithm is as follows :
- Generate possible solutions.
- Test to see if this is the expected solution.
- If the solution has been found quit else go to step 1.
Hence we call Hill climbing a variant of generating and test algorithm as it takes the feedback from the test procedure. Then this feedback is utilized by the generator in deciding the next move in the search space.
- Uses the Greedy apporach:
At any point in state space, the search moves in that direction only which optimizes the cost of function with the hope of finding the optimal solution at the end.
Adversarial Search
Adversarial search is a search, where we examine the problem which arises when we try to plan ahead of the world and other agents are planning against us.In previous topics, we have studied the search strategies which are only associated with a single agent that aims to find the solution which often expressed in the form of a sequence of actions.
But, there might be some situations where more than one agent is searching for the solution in the same search space, and this situation usually occurs in game playing.
The environment with more than one agent is termed as multi-agent environment, in which each agent is an opponent of other agent and playing against each other. Each agent needs to consider the action of other agent and effect of that action on their performance.
Types of Games in AI:
Perfect information: A game with the perfect information is that in which agents can look into the complete board. Agents have all the information about the game, and they can see each other moves also. Examples are Chess, Checkers, Go, etc.
Imperfect information: If in a game agents do not have all information about the game and not aware with what’s going on, such type of games are called the game with imperfect information, such as tic-tac-toe, Battleship, blind, Bridge, etc.
Deterministic games: Deterministic games are those games which follow a strict pattern and set of rules for the games, and there is no randomness associated with them. Examples are chess, Checkers, Go, tic-tac-toe, etc.
Non-deterministic games: Non-deterministic are those games which have various unpredictable events and has a factor of chance or luck. This factor of chance or luck is introduced by either dice or cards.
These are random, and each action response is not fixed. Such games are also called as stochastic games.
Example: Backgammon, Monopoly, Poker, etc
Zero-Sum Game :-Zero-sum games are adversarial search which involves pure competition.In Zero-sum game each agent’s gain or loss of utility is exactly balanced by the losses or gains of utility of another agent.
One player of the game try to maximize one single value, while other player tries to minimize
Zero-sum game: Embedded thinkingThe Zero-sum game involved embedded thinking in which one agent or player is trying to figure out:
- What to do.
- How to decide the move
- Needs to think about his opponent as well
- The opponent also thinks what to do
Game tree:
A game tree is a tree where nodes of the tree are the game states and Edges of the tree are the moves by players. Game tree involves initial state, actions function, and result Function.Example: Tic-Tac-Toe game tree:
The following figure is showing part of the game-tree for tic-tac-toe game. Following are some key points of the game:
- There are two players MAX and MIN.
- Players have an alternate turn and start with MAX.
- MAX maximizes the result of the game tree
- MIN minimizes the result.
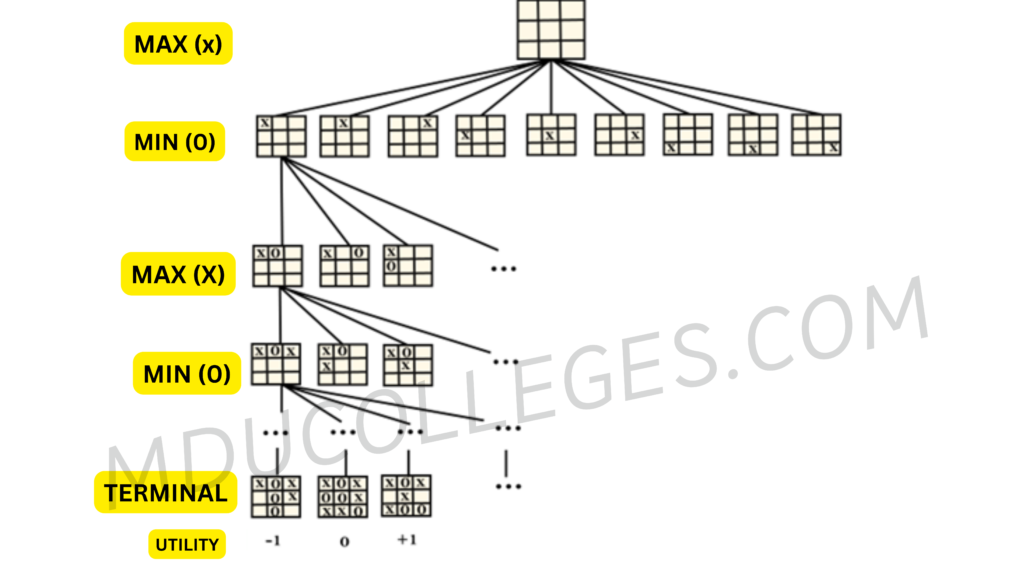
Mini-Max Algorithm in Artificial Intelligence
- Mini-max algorithm is a recursive or backtracking algorithm which is used in decision-making and game theory. It provides an optimal move for the player assuming that opponent is also playing optimally.
- Mini-Max algorithm uses recursion to search through the game-tree.
- Min-Max algorithm is mostly used for game playing in AI. Such as Chess, Checkers, tic-tac-toe, go, and various tow-players game. This Algorithm computes the minimax decision for the current state.
- In this algorithm two players play the game, one is called MAX and other is called MIN.
- Both the players fight it as the opponent player gets the minimum benefit while they get the maximum benefit.
- Both Players of the game are opponent of each other, where MAX will select the maximized value and MIN will select the minimized value.
Pseudo-code for MinMax Algorithm:
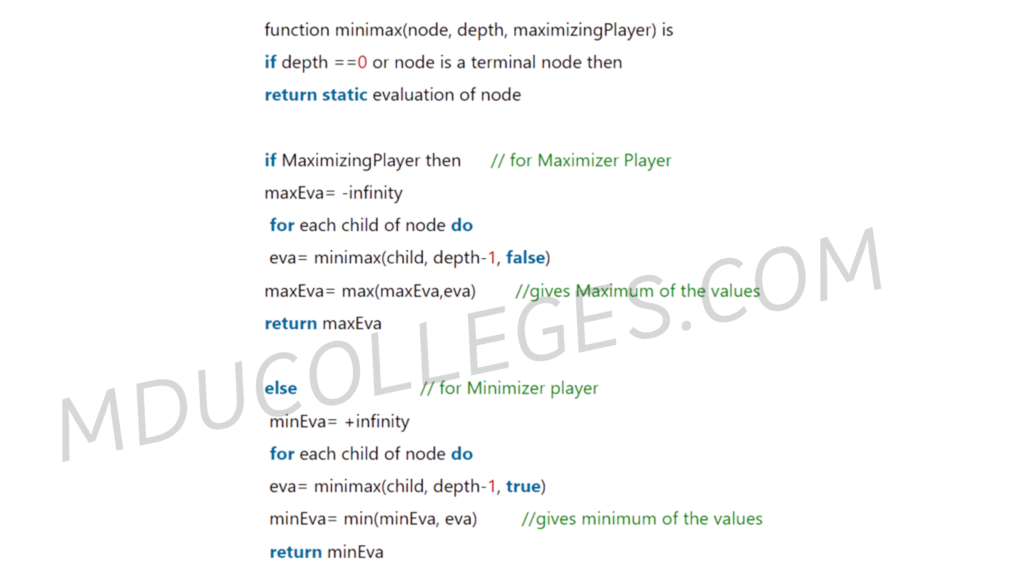
Working of Min-Max Algorithm:
- The working of the minimax algorithm can be easily described using an example. Below we have taken an example of game-tree which is representing the two-player game.
- In this example, there are two players one is called Maximizer and other is called Minimizer.
- Maximizer will try to get the Maximum possible score, and Minimizer will try to get the minimum possible score.
- This algorithm applies DFS, so in this game-tree, we have to go all the way through the leaves to reach the terminal nodes.
- At the terminal node, the terminal values are given so we will compare those value and backtrack the tree until the initial state occurs. Following are the main steps involved in solving the two-player game tree:
Step-1: In the first step, the algorithm generates the entire game-tree and apply the utility function to get the utility values for the terminal states. In the below tree diagram, let’s take A is the initial state of the tree. Suppose maximizer takes first turn which has worst-case initial value =- infinity, and minimizer will take next turn which has worst-case initial value = +infinity.
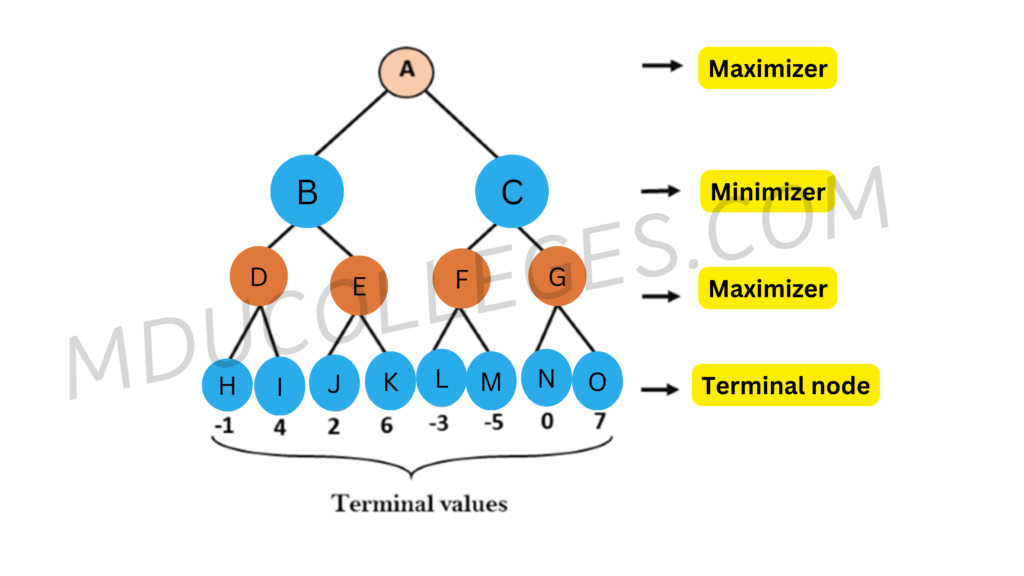